As a startupper, you are no stranger to the relentless pressures of competition, market volatility, and the unyielding demand for innovative solutions. You might think that the challenge of staying with the trends while delivering exceptional value to your users is insurmountable. Traditional trading strategies, once reliable, are now being outpaced by cutting-edge technologies and ever-evolving market dynamics, such as AI.
However, how can you leverage smart algorithms for new pathways to profitability, enhanced decision-making, and automated efficiency? Should you opt for them instead of offering traditional methods? Won’t you sacrifice your unique value proposition?
We will dive deep into the transformative role of AI in stock trading, unpacking the tools and strategies that can empower your startup to thrive in this competitive arena. With insights tailored for agile mindsets, we’ll explore the solutions that could redefine your trading strategy and set your startup apart from the competitors.
What is AI in Stock Trading
These are computer programs and algorithms that can analyze large amounts of data to make decisions about buying and selling stocks. They identify patterns, predict price movements, and execute trades automatically, often faster and more efficiently than a human trader.
AI isn’t just a buzzword now—it’s a critical tool for fintech startups looking to revolutionize how trading is conducted. With the help of Artificial Intelligence, your startup can optimize trading strategies, improve risk management, personalize user experiences, and ultimately position itself as a leader in the fintech space.
Evolution of Trading
From Manual to AI
How did we get from traditional methods to smart algorithms? The evolution of stock trading has changed a lot over time.
Before AI, stock market trading was one hundred percent manual, done by people on the trading floor. They would shout orders and write them down on paper. The approach relied heavily on personal knowledge and intuition.
Then, traders began using telephones and faxes to place orders, making communication faster and easier but still requiring lots of manual effort.
With the rise of computers in the late 20th century, stock trading moved online. Investors could buy and sell stocks through websites, making them more accessible to the public and speeding up the process.
As technology advanced, people started using algorithms—sets of rules programmed into computers to make trades automatically. These algorithms could analyze data much faster than humans and execute trades in milliseconds.
Now, we are in the AI era, where machine learning techniques allow computers to analyze huge amounts of market data. These systems can spot complex patterns and make predictions, helping businesses optimize their strategies even more efficiently.
Human vs AI in Stock Market
The fintech evolution picked AI-powered methods because they offer distinct advantages over traditional ones. As well as challenges.
Decision-Making Speed
AI can process vast amounts of data and execute trades in milliseconds, allowing for rapid reaction to market changes.
Human decision-making is slower as it relies on cognitive processing and emotional factors, which can lead to delayed reactions in fast-moving markets.
Data Analysis
AI in stock market utilizes machine learning algorithms to analyze historical and real-time data, uncovering patterns and trends that may not be visible at a glance.
While people can interpret qualitative data and broader market sentiment, they can’t analyze large datasets as efficiently as AI does.
Emotional Factors
Without emotions, artificial intelligence makes more rational decisions and is unaffected by fear or greed.
Humans are prone to emotional biases that can cloud judgment, potentially resulting in impulsive decisions or overtrading during volatility.
Adaptability
Machine learning algorithms learn and adapt to new market conditions, but rely on historical data for training and may struggle with unprecedented events.
People use intuition and reasoning to adapt to rapidly changing or unique market scenarios.
Cost and Efficiency
Once developed, algorithms can execute trades at a low cost and operate continuously without fatigue.
People are no machines and require significant time and mental energy. Without rest, they often suffer from burnout, which affects performance over time.
Regulatory Challenges
AI in stock trading has to be programmed correctly and comply with financial regulations. Without restrictions, it may inadvertently engage in practices like market manipulation.
A human faces regulatory scrutiny, however, personal accountability ensures adherence to ethical trading practices.
Flexibility in Strategy
Limited by its programming, Artificial Intelligence may require significant adjustments or retraining to shift strategies.
People can quickly pivot and adapt strategies based on new information, intuition, or changing market conditions.
As you can see, both human and AI stock market trading have their own strengths and weaknesses. For now, the optimal approach involves a hybrid model, leveraging AI’s analytical power alongside human insight and intuition to navigate the complexities of the market effectively.
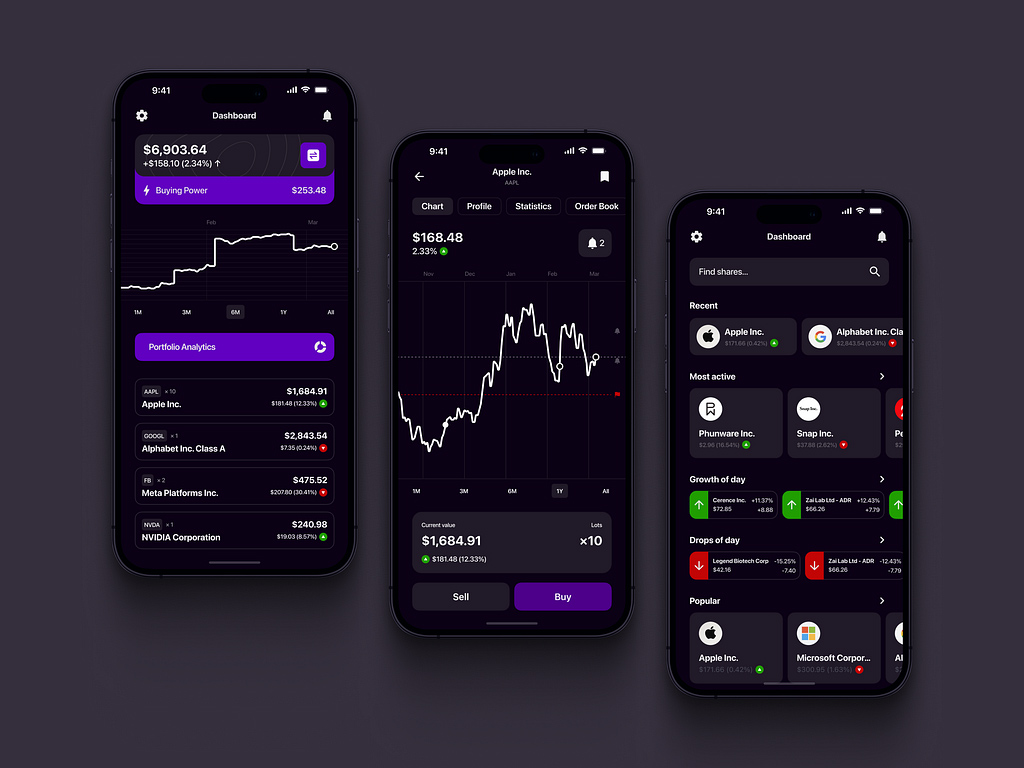
Stocks Trading Mobile App by Conceptzilla
Key AI Technologies Transforming the Industry
These tools are revolutionizing stock trading, enabling greater efficiency, accuracy, and insights, and changing the way players operate in financial markets.
- Machine Learning: Algorithms analyze historical market data to identify patterns and predict future price movements.
- Natural Language Processing: You can analyze news articles, financial reports, and social media sentiment to gauge market trends and investor sentiment.
- Algorithmic Trading: Using AI for stock trading, you execute trades based on pre-set rules and conditions, allowing for high-frequency trading and the ability to manage large volumes of transactions automatically.
- Predictive Analytics: Utilize statistical models and machine learning to forecast market trends and price movements, helping users make informed decisions.
- Sentiment Analysis: Evaluate the market mood by analyzing public sentiment on social media, news feeds, and forums to predict market reactions.
- Robo-Advisors: Smart assistants provide automated, algorithm-driven financial planning services with minimal human intervention, managing portfolios based on user risk profiles.
- Portfolio Management: These tools optimize investment portfolios by continuously analyzing asset performance and market conditions to adjust holdings dynamically.
- Risk Management: AI technologies analyze various risk factors and provide insights into potential market disruptions, helping you mitigate risks effectively.
- Backtesting: With algorithms, you can simulate trading strategies against historical data to evaluate their effectiveness before being applied in real-time trading scenarios.
- Neural Networks: Artificial Intelligence mimics human brain functions to identify complex patterns in data, enhancing predictive accuracy for stock prices.
How to Use AI in Stock Trading Apps
Using AI in stock trading apps can significantly enhance trading strategies, improve decision-making, and optimize portfolio management. If you decide to leverage smart algorithms for your fintech app, then there are several steps you need to take:
- Define Objectives: Determine the purpose of the AI integration, such as predictive analytics, risk assessment, or algorithmic trading.
- Data Acquisition: Gather and process large datasets from various sources (market data, news articles, social media). Include external data like news sentiment, economic indicators, and social media trends.
- Data Processing: Clean and preprocess data to remove noise and ensure consistency. Normalize and scale data for better model performance.
- Choose the Right Tools: Explore various machine learning models. For example, regression models for price prediction (e.g., Linear Regression, Random Forest), time series analysis (e.g., ARIMA, LSTM) for forecasting stock trends, or reinforcement learning for developing trading strategies.
- Model Training: Split the dataset into training and testing sets. Train models using historical data and validate their performance through backtesting.
- Optimization: Optimize model parameters to enhance prediction accuracy. Use techniques like cross-validation to avoid overfitting.
- Deployment: Integrate the trained model into your stock trading app. Implement real-time data feeds to enable live predictions.
- Monitoring & Updating: Continuously monitor model performance and market conditions. Regularly update models with new data to adapt to changing market dynamics.
Case Studies of Successful Startups
At Shakuro, we also had an opportunity to work on several fintech projects that were using AI for stock trading.
Korean Trading App
Solio is aimed at beginner traders. Since the target audience has little experience, users need guidance until they can make their own decisions. That’s why we’ve designed a friendly robo-advisor that analyzes the user portfolio and provides valuable insights. With its help, users can manage their finances in an efficient and stressless way.
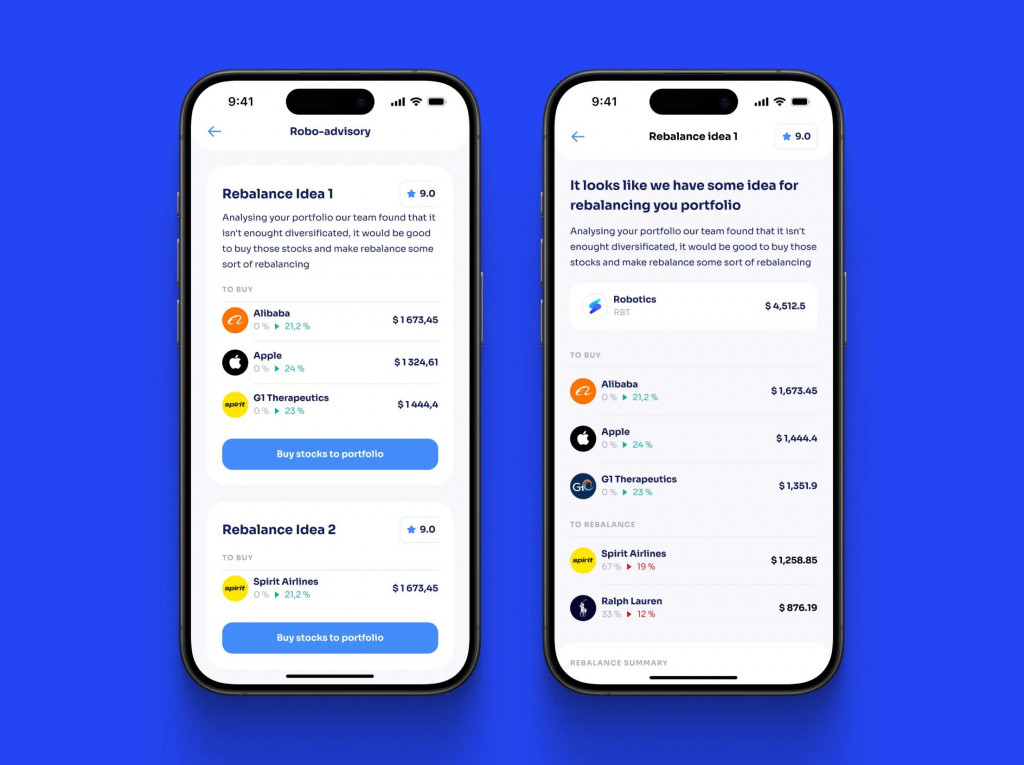
Solio app by Shakuro
Mobile Investment Platform
One of the challenges here was implementing all cutting-edge tools while complying with Sharia laws—the app targeted investors with little experience in the Arabic market. To cater to their needs, our team combined robo-advisor with professional trading features. The smart assistant gives people a firm footing while they gain enough knowledge to make their own steps.
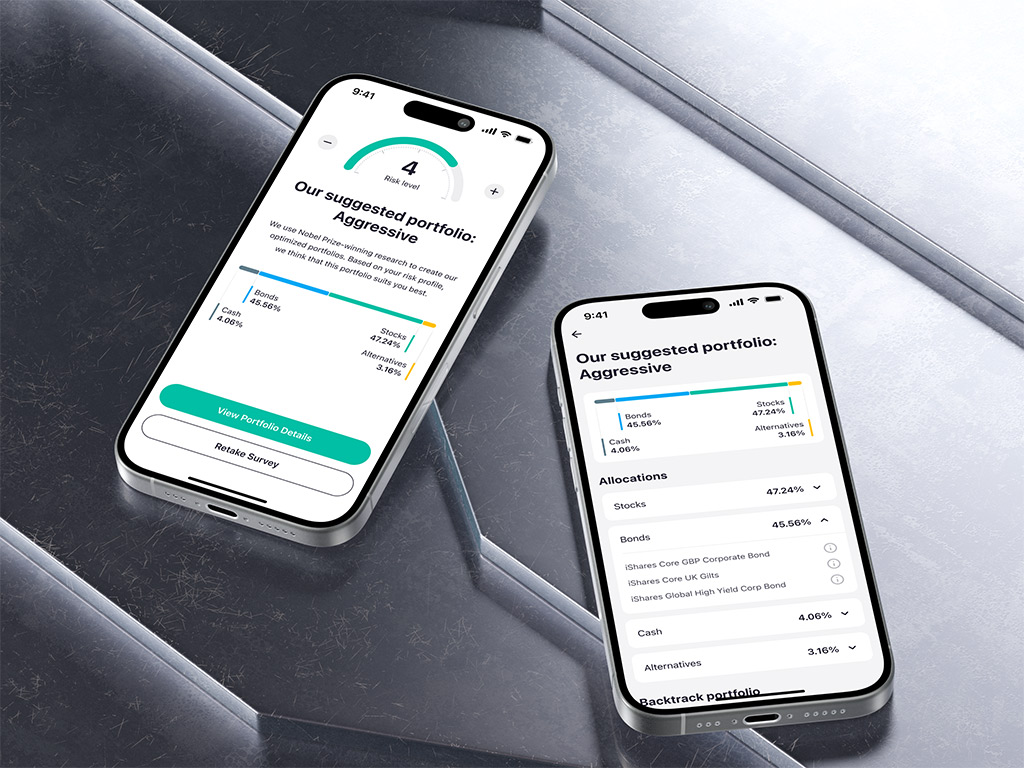
Risk profiling in ZAD app
Financial Analytics Tool
This is a more complex tool featuring the latest advancements in AI—it offers large language models (LLMs) like ChatGPT to expand its capabilities and enhance the user experience. With their help, traders can quickly analyze complex financial documents such as annual reports, earnings call transcripts, and regulatory filings. Professionals can customize AI models according to their specific requirements, allowing for greater relevance and precision in AI-generated insights.
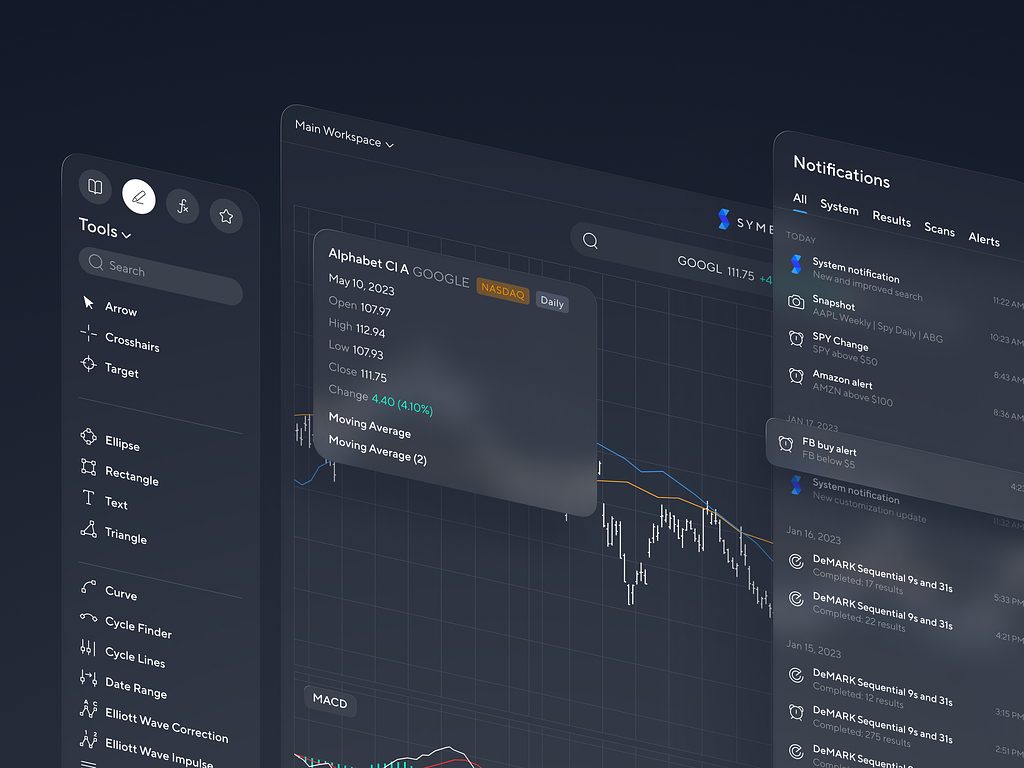
Stock Market Analytics Application by Shakuro
Conclusion
Now that you know how to use AI in stock trading and how it impacts the market. It is transformative, ushering in a new era of efficiency and insight.
While traditional trading methods are not being rendered obsolete, the integration of AI is elevating the game for those willing to adapt. As we move forward, the challenge will be to balance human intuition with machine precision, creating a hybrid approach that leverages the strengths of both.
Do you need a development team to help you integrate AI in stock trading apps? Reach out to us and let’s start working together on your future projects.