In the bustling arena of e-commerce, you often find yourself on an exhilarating yet daunting journey. The excitement of launching a new venture is matched only by the relentless challenges of standing out in a crowded digital marketplace.
Picture this: a tool that understands your customers better than you do, a tool that not only anticipates their needs but also has the power to make recommendations that drive sales and elevate your brand to unprecedented heights.
This tool is an AI recommender system, and in the world of e-commerce, it’s the beacon of hope that can transform your startup into a thriving enterprise. In this article, we will take you on a journey through the possibilities, strategies, and benefits of building an AI recommendation system tailored for your e-commerce business. Prepare to embark on a game-changing adventure that promises to alleviate your startup’s pains, boost your revenue, and propel you toward success.
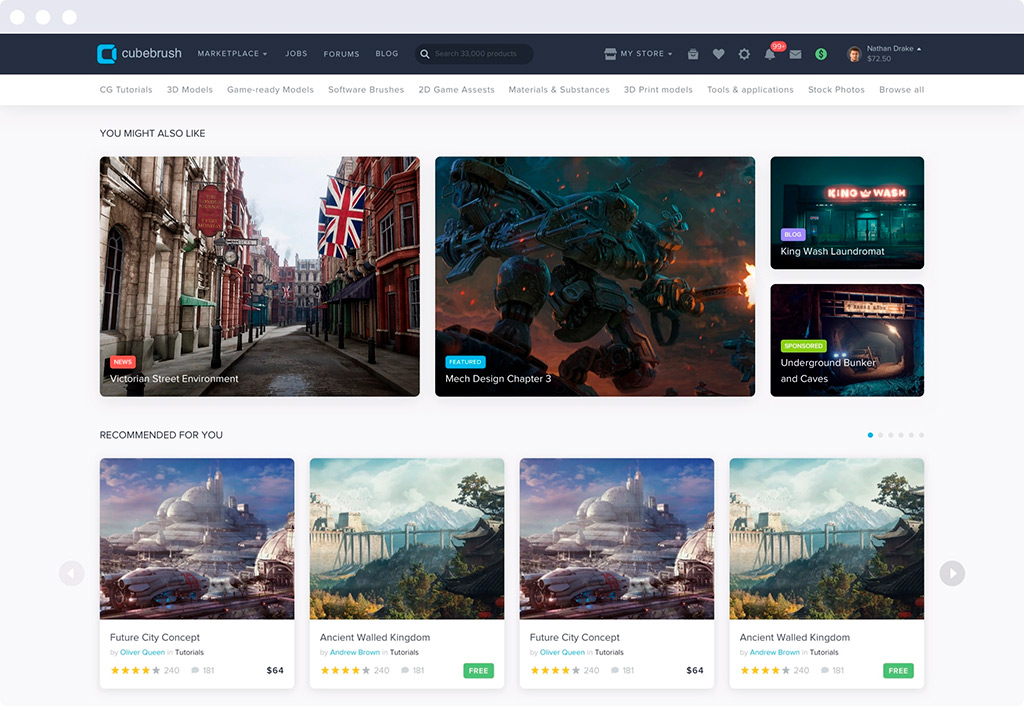
Recommendations in one of the platforms we created, Cubebrush
What is a recommendation engine
It is an application that uses data and algorithms to provide personalized product or content recommendations to users. Remember, when you enter an online shop, do you often see a section named you-might-also-like? That’s the e-commerce recommendation. The primary goal is to enhance the UX by suggesting items that align with a user’s preferences, needs, and behaviors, ultimately increasing engagement and sales.
How does it work?
Here’s how recommendation engine algorithms in e-commerce typically work:
- Data collection: First, the system collects and aggregates data from various sources, including user interactions (e.g., clicks, purchases, searches), profiles, product information, and more.
- Data processing: Then it cleans the gathered data, processes and transforms it into a format suitable for analysis.
- Analysis: The engine applies advanced algorithms and machine learning models to identify patterns, correlations, and user preferences. Common recommendation algorithms include collaborative filters, content-based filtering, hybrid models, and deep learning techniques.
- User profiling: The system creates individual user profiles based on their past interactions and behavior. They include information about their preferences, interests, and demographics.
- Recommendation generation: Using the insights gained from data analysis, the recommendation engine creates personalized suggestions for products, services, or content. It can be product suggestions, related items, content recommendations, or targeted promotions.
- Presentation: The system can present recommended items through various channels, including the e-commerce website, mobile apps, emails, or even chatbots. The key is to integrate the section with the user interface seamlessly.
- Feedback loop: If the user doesn’t engage with suggested items, the system refines its recommendations to improve their relevance over time.
Types of product recommendation engines
We mentioned them above briefly but now let’s dwell on each type since they have their own strengths and use cases:
- Collaborative filters: This technique recommends items based on the preferences and behaviors of users with similar profiles. It’s effective for suggesting items that others with similar tastes have liked.
- Content-based filtering: it matches the content or product to the user’s preferences. For example, recommending a dress in a similar style to one a person previously purchased.
- Hybrid recommender systems: they combine two previous systems to provide more accurate and diverse suggestions.
- Deep learning models: neural networks process large amounts of data and can capture intricate patterns and relationships, making them suitable for complex recommendation tasks.
Potential benefits of algorithmic recommendations
For startups, the benefits of implementing AI in e-commerce are substantial. These advantages include:
Increased sales and revenue
When you suggest products that align with customer preferences, your clients are more likely to make a purchase. So you boost sales and revenue.
Enhanced customer engagement
Personalized recommendations keep customers engaged and coming back for more. It is a key driver of customer satisfaction and loyalty. When customers feel understood and catered to, they are more likely to spend time exploring your offerings.
Improved customer retention
AI-driven algorithmic recommendations not only drive initial sales but also keep customers returning for repeat purchases. This can significantly improve customer retention rates.
Competitive advantage
When starting a business, you often struggle to compete with established e-commerce giants. AI recommendation systems can help you level the playing field by providing a unique and compelling user experience.
Efficient inventory management
With smart suggestions, you manage inventory more efficiently by predicting demand trends, reducing overstocking or understocking issues, and optimizing product listings.
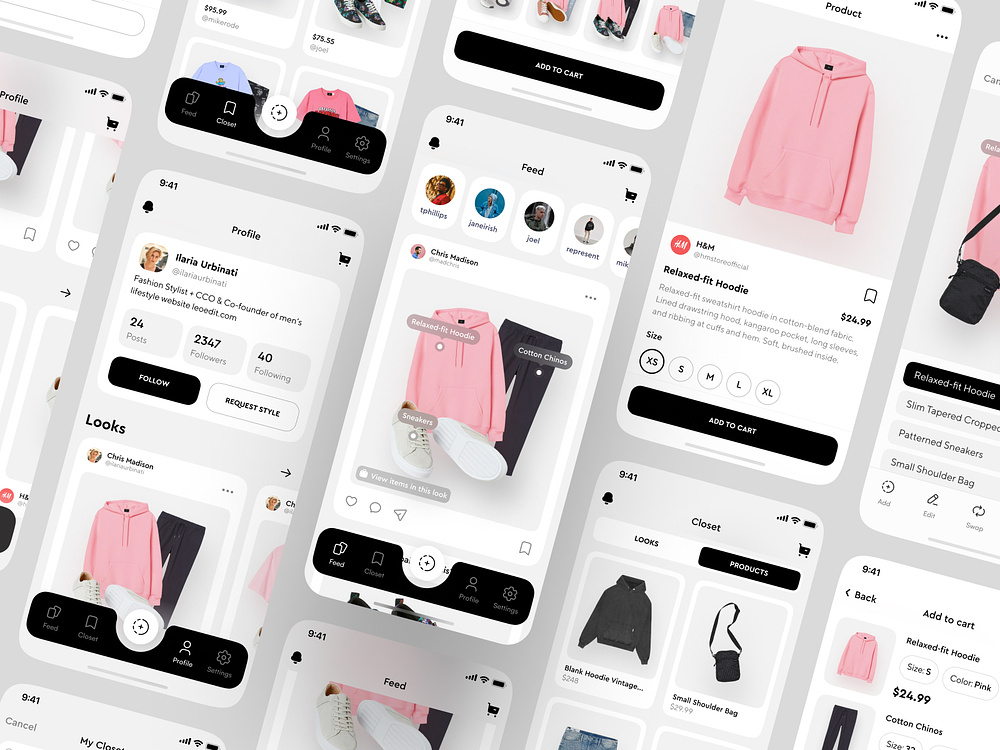
Wear Crocks to a Theater by Shakuro
How to implement AI product recommendation engines in your startup
Begin by defining what you want to achieve with recommendations. Are you aiming to increase sales, improve customer retention, enhance user engagement, or all of the above? Having clear goals will guide your implementation strategy.
Then gather and clean the necessary data, including customer behavior information (clicks, purchases, searches), profiles, and product catalog information. Make sure that your data is accurate, complete, and properly structured.
Once the data is prepared, choose the recommendation engine that best suits your startup’s needs. The choice depends on your data, available resources, and goals.
Integrate the system with your e-commerce platform. Depending on your technology stack, this may involve custom development or the use of third-party solutions and APIs. If you’re considering this approach, thoroughly evaluate potential vendors to ensure they align with your startup’s needs and goals.
The design is also important here. Recommendations should enhance the user experience without being intrusive. Ensure that the new section fits into your website or app’s interface.
Implement A/B testing to compare the performance of your algorithmic recommendations against non-personalized content. Continuously monitor key performance metrics, such as click-through rates, conversion rates, and revenue, and use the insights gained to optimize the system.
If you want to dive deeper into integrating AI into your e-commerce store, read our interview with the team lead about using ChatGPT. You will find tips for creating personalized recommendations, letters, products, etc.
Overcoming the challenges
Implementing AI in e-commerce can be highly beneficial for your business, but it also comes with its fair share of challenges. Here are some common pitfalls you might face:
- Data quality: insufficient or low-quality data can hinder the effectiveness of your recommendation system. You need to have enough high-quality data to train your algorithms.
- Algorithm cold start: when you have limited user interaction info, such as for new, generating relevant suggestions can be difficult. Handling these “cold start” scenarios is a challenge.
- Cold-start products: the same goes for new products added to your catalog. They have limited data for recommendations, making it challenging to promote them effectively.
- Privacy and security: collecting and analyzing user data raises privacy concerns. So make sure to follow data privacy regulations like GDPR. Moreover, it’s wise to invest in robust security features.
- Scalability: as your e-commerce platform grows, your recommender system must handle increasing data volumes and user traffic without spilling bugs. And scaling the system can be a complex task.
- Algorithm accuracy: ensuring that your recommendation algorithms provide relevant and accurate suggestions requires continuous monitoring and optimization.
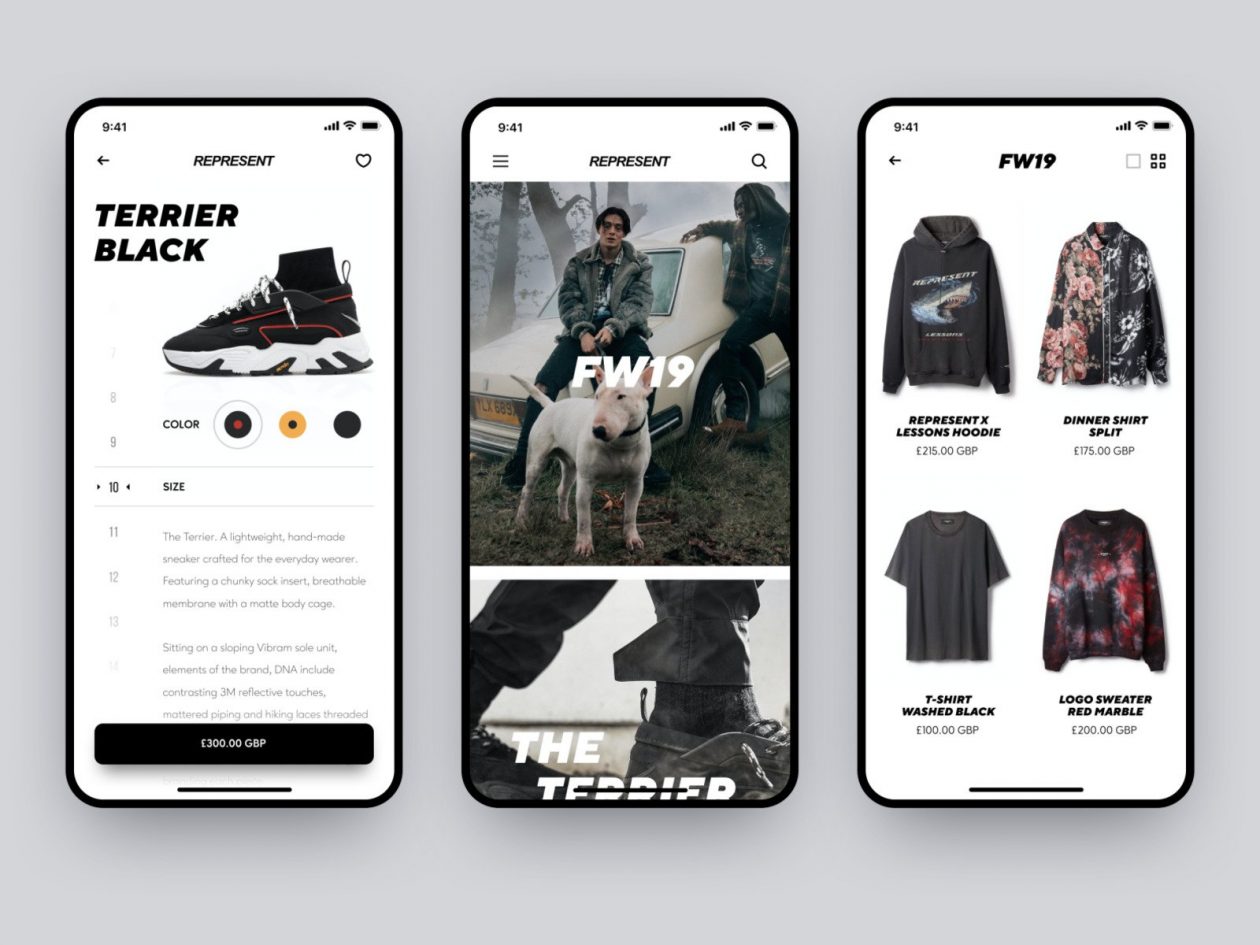
Represent App Concept by Shakuro
Tips for choosing the right AI e-commerce recommendation engine
Trial period
Opt for a trial period to test the solution’s effectiveness within your specific e-commerce environment before committing to a long-term contract.
Customization options
Evaluate whether the solution allows for customization to align with your project’s unique requirements and target audience pains.
Compatibility
The AI e-commerce solution you select should be compatible with your existing technology stack, including your e-commerce platform, databases, and other tools.
Budget considerations
Consider both the upfront costs and long-term ROI when budgeting for an AI recommendation system.
Conclusion
In conclusion, e-commerce recommendation systems hold immense potential for startups looking to succeed in the competitive world of online retail. You can leverage AI and smart suggestions to create personalized shopping experiences, boost sales, and gain a competitive edge.
Does your project require a functional e-commerce platform with relevant product recommendations? Reach out to us and let’s build a website that boosts your business and sales.